Machine Learning, Biased Models, and Finding the Truth
Esther Shein | 27 November 2018
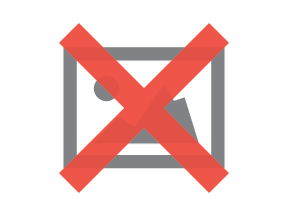
Patrick Ball, Director of Research, Human Rights Data Analysis Group, offered examples of when statistics and machine learning have proved useful and when they’ve failed in this presentation from Open Source Summit Europe.
Machine learning and statistics are playing a pivotal role in finding the truth in human rights cases around the world – and serving as a voice for victims, Patrick Ball, director of Research for the Human Rights Data Analysis Group, told the audience at Open Source Summit Europe.
Ball began his keynote, “Digital Echoes: Understanding Mass Violence with Data and Statistics,” with background on his career, which started in 1991 in El Salvador, building databases. While working with truth commissions from El Salvador to South Africa to East Timor, with international criminal tribunals as well as local groups searching for lost family members, he said, “one of the things that we work with every single time is trying to figure out what the truth means.”
In the course of the work, “we’re always facing people who apologize for mass violence. They tell us grotesque lies that they use to attempt to excuse this violence. They deny that it happened. They blame the victims. This is common, of course, in our world today.”
Human rights campaigns “speak with the moral voice of the victims,’’ he said. Therefore, it is critical that statistics, including machine learning, are accurate, Ball said.
He gave three examples of when statistics and machine learning proved to be useful, and where they failed.
Finding missing prisoners
In the first example, Ball recalled his participation as an expert witness in the trial of a war criminal, the former president of Chad, Hissène Habré. Thousands of documents were presented, which had been discovered as a pile of trash in an abandoned prison and which turned out to be the operational records of the secret police.
The team honed in one type of document that detailed the number of prisoners that were held at the beginning of the day, the number held at the end of the day, and the difference between the number of prisoners who were released, new prisoners brought in, those transferred to other places, and those who had died during the course of the day. Dividing the number of people who died throughout the day by the number alive in the morning produces the crude mortality rate, he said.
The status of the prisoners of war was critical in the trial of Habré because the crude mortality rate was “extraordinarily high,” he said.
“What we’re doing in human rights data analysis is … trying to push back on apologies for mass violence. In fact, the judges in the [Chad] case saw precisely that usage and cited our evidence … to reject President Habré’s defense that conditions in the prison were nothing extraordinary.”
That’s a win, Ball stressed, since human rights advocates don’t see many wins, and the former head of state was sentenced to spend the rest of his life in prison.
Hidden graves in Mexico
In a more current case, the goal is to find hidden graves in Mexico of the bodies of people who have disappeared after being kidnapped and then murdered. Ball said they are using a machine learning model to predict where searchers are likely to find those graves in order to focus and prioritize searches.
Since they have a lot of information, his team decided to randomly split the cases into test and training sets and then train a model. “We’ll predict the test data and then we’ll iterate that split, train, test process 1,000 times,’’ he explained. “What we’ll find is that over the course of four years that we’ve been looking at, more than a third of the time we can perfectly predict the counties that have graves.”
“Machine learning models are really good at predicting things that are like the things they were trained on,” Ball said.
A machine learning model can visualize the probability of finding mass graves by county, which generates press attention and helps with the advocacy campaign to bring state authorities into the search process, he said.
That’s machine learning, contributing positively to society,” he said. Yet, that doesn’t mean that machine learning is necessarily positive for society as a whole.
Predictive Policing
Many machine learning applications “are terribly detrimental to human rights and society,’’ Ball stressed. In his final example, he talked about predictive policing, which is the use of machine learning patterns to predict where crime is going to occur.
For example, Ball and his team looked at drug crimes in Oakland, California. He displayed a heat map of the density of drug use in Oakland, based on a public health survey, showing the highest drug use close to the University of California.
Ball and his colleagues re-implemented one of the most popular predictive policing algorithms to predict crimes based on this data. Then he showed the model running in animation, with dots on the grid representing drug arrests. Then the model made predictions in precisely the same locations as where the arrests were observed, he said.
If the underlying data turns out to be biased, then “we recycle that bias. Now, biased data leads to biased predictions.” Ball went on to clarify that he was using the term bias in a technical, not racial sense.
When bias in data occurs, he said, it “means that we’re over predicting one thing and that we’re under predicting something else. In fact, what we’re under predicting here is white crime,’’ he said. Then the machine learning model teaches police dispatchers that they should go to the places they went before. “It assumes the future is like the past,” he said.
“Machine learning in this context does not simply recycle racial disparities in policing, [it] amplifies the racial disparities in policing.” This, Ball said, “is catastrophic. Policing already facing a crisis of legitimacy in the United States as a consequence of decades, or some might argue centuries, of unfair policing. ML makes it worse.”
“In predictive policing, a false positive means that a neighborhood can be systematically over policed, contributing to the perception of the citizens in that neighborhood that they’re being harassed. That erodes trust between the police and the community. Furthermore, a false negative means that police may fail to respond quickly to real crime,” he said.
When machine learning gets it wrong
Machine learning models produce variances and random errors, Ball said, but bias is a bigger problem. “If we have data that is unrepresentative of a population to which we intend to apply the model, the model is unlikely to be correct. It is likely to reproduce whatever that bias is in the input side.”
We want to know where a crime has occurred, “but our pattern of observation is systematically distorted. It’s not that [we] simply under-observe the crime, but under-observe some crime at a much greater rate than other crimes.” In the United States, he said, that tends to be distributed by race. Biased models are the end result of that.
The cost of a machine learning being wrong can also destroy people’s lives, Ball said. It also raises the question of who bears the cost of being wrong. You can hear more from Ball and learn more about his work in the complete video presentation below.
Similar Articles
Browse Categories
Cloud Computing Compliance and Security Open Source Projects 2024 LF Research Blog Linux How-To Open Source Ecosystem and Governance Diversity & Inclusion Research Newsletter Data, AI, and Analytics linux blog Linux Training and Certification Cross Technology software development Cloud Native Computing Foundation cybersecurity lf events Announcements Decentralized Technology Legal OpenSearch Sustainability and Green Initiatives cloud native generative AI industries Finance and Business Technology Networking and Edge cncf AI/ML Emerging Technology Health and Public Sector Interoperability Kubernetes Topic: Security Web Application & Development amazon web services aws careers community tools confidential computing challenges decentralized AI decentralized computing eBPF education funding innovation investment japan spotlight kernel learning lg blog license compliance open standards openssf ospo project news research survey skills development state of open source tech talent transformation